You can find this article here: https://medium.com/doctrine/scaling-with-postgresql-50e6de647acf
Scaling with PostgreSQL
Yes you can scale PostgreSQL! And this is how we did it at Doctrine.
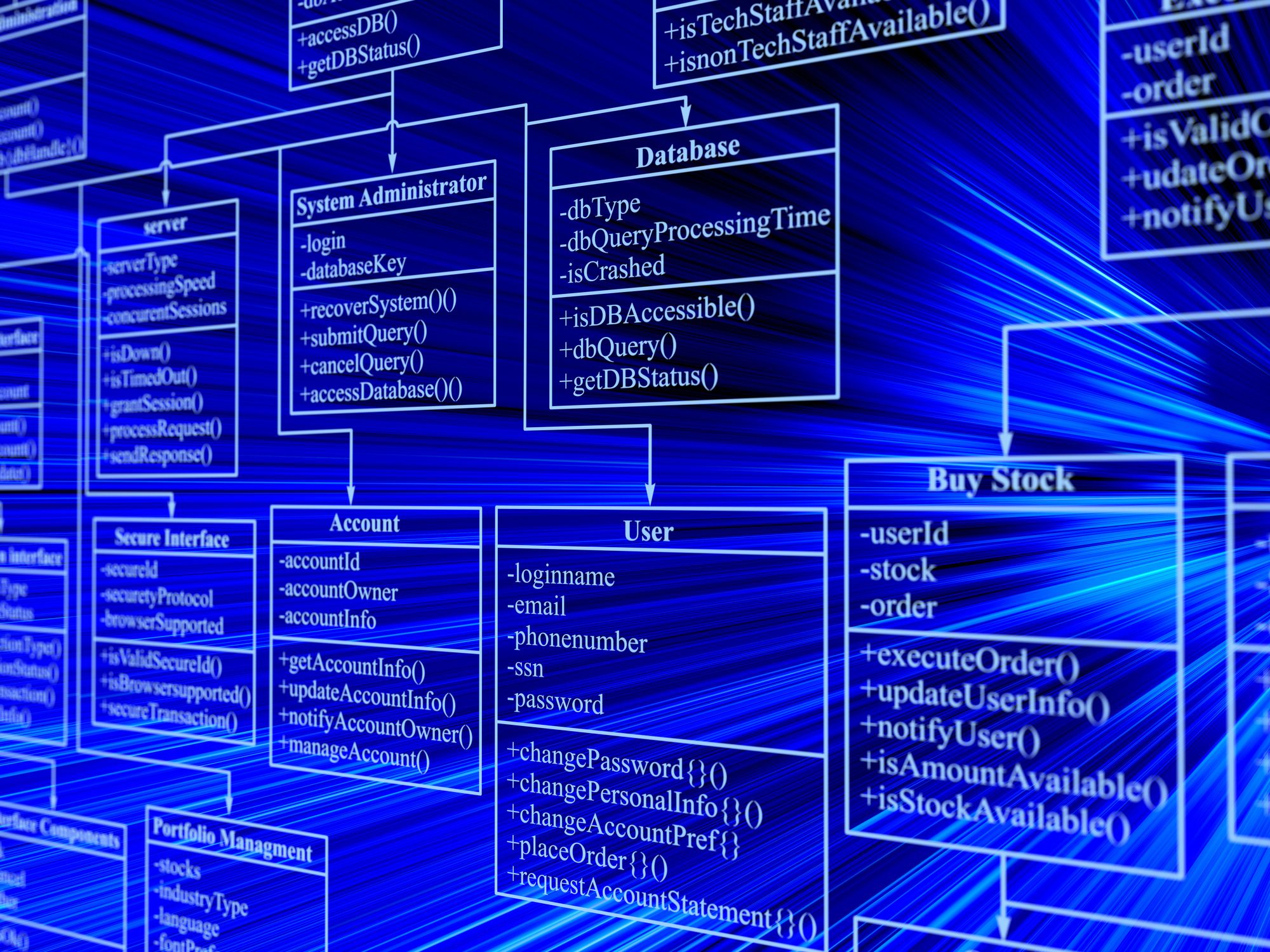
Subscribe to Doctrine le Blog
Get the latest posts delivered right to your inbox